SSRP Abstract
Inference for Regressional Model Coefficients When Data Has Been Subjected to Censoring
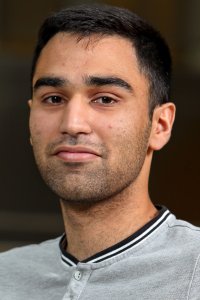
Student: Muzaffar Yezdan ’22
Research Mentor: Scott Linder (OWU Department of Mathematics and Computer Science)
Most clinical and industrial trials are subjected to a phenomenon known as censoring, which leads to the data collected from the trial to be incomplete. This missing data makes it difficult to infer information about the population based on the small-scale trial. We looked into methods of making more accurate statistical inferences from censored data which would allow researchers to more effectively utilize data collected from trials.
Clinical and industrial trials offer crucial insights into how well drugs or electronic components perform. Data from these trials is sometimes subjected to censoring — clinicians stop the experiment at a certain time or after a predetermined number of observations has been made. When data have been subjected to censoring, the sampling distributions of certain statistics computed from the data are mathematically intractable, making statistical inference difficult. In this work we consider the sampling distribution of certain regression-associated sums of squares used to estimate variance. Using simulation, we demonstrate that the sampling distributions of these statistics are impacted by censoring, and differ from those one would use for full (uncensored) samples. We propose approximating these sampling distributions by Gamma distributions with parameters that are functions of the experimental conditions (sample size and degree of censoring). We then examine the performance of confidence intervals for population variances that arise from this approximation.